Bayesian Hierarchical Models of Presidential Election Polling
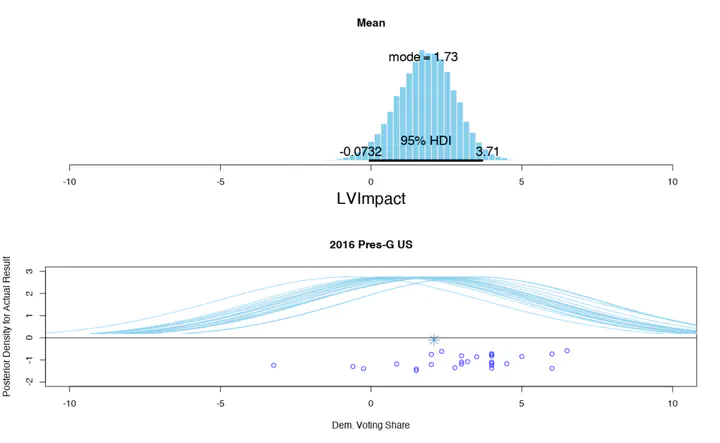
In the lead up to any major American election, many pollsters conduct surveys to estimate the amount of support each candidate has. Pollsters have to make a number of decisions that impact the accuracy of their polls, such as estimating which of their poll respondents will actually turn out for the election. Every pollster approaches these questions differently, and consequently even polls conducted around the same time can give contradictory estimates!
I work on constructing statistical models that take these polls and try to make sense of the contradictory information. Each poll will miss the true level of support for a candidate for a variety of reasons, such as random sampling error or systematic pollster bias. Our hierarchical models try to account for these potential sources of error using features of the poll such as the type of voter model employed and the past biases of the pollster.
The overarching goal is to construct a Bayesian model for election forecasting similar to those developed at FiveThirtyEight and The Economist to understand the dynamics of American elections.
Collaborators:
- Haley Reed (‘22), Summer 2020
Future Projects:
- Further develop hierarchical pollster model
- Integrate time series modeling to forecast the current state forward
- Develop data science pipeline to aggregate and annotate a wide variety of polls